This abstract explores the paradigm of a forward-looking approach to fortifying network security in the dynamic landscape of cyber threats. The study investigates the integration of adaptive defense strategies, leveraging the capabilities of machine learning algorithms to dynamically respond to evolving cyber risks. By continuously learning from real-time data, the proposed system adapts its defense mechanisms to emerging threats, providing a proactive and resilient network security posture. The abstract emphasizes the significance of adaptability in mitigating sophisticated attacks, highlighting the effectiveness of machine learning algorithms in detecting, preventing, and responding to security incidents. Through this adaptive defense framework, organizations can foster a robust and agile security infrastructure that anticipates and counteracts cyber threats with a high degree of precision and efficiency.
Adaptive Defense: Enhancing Network Security through Machine Learning Algorithms
Publication Information
Journal Title: Asian Journal of Multidisciplinary Research & Review
Author(s): Danny Jhonson & Jane Smith
Published On: 05/03/2024
Volume: 5
Issue: 1
First Page: 134
Last Page: 145
ISSN: 2582-8088
Publisher: The Law Brigade Publisher
Cite this Article
Danny Jhonson & Jane Smith, Adaptive Defense: Enhancing Network Security through Machine Learning Algorithms, Volume 5 Issue 1, Asian Journal of Multidisciplinary Research & Review, 134-145, Published on 05/03/2024, 10.55662/AJMRR.2024.5104 Available at https://ajmrr.thelawbrigade.com/article/adaptive-defense-enhancing-network-security-through-machine-learning-algorithms/
Abstract
Share this research
Latest Publications
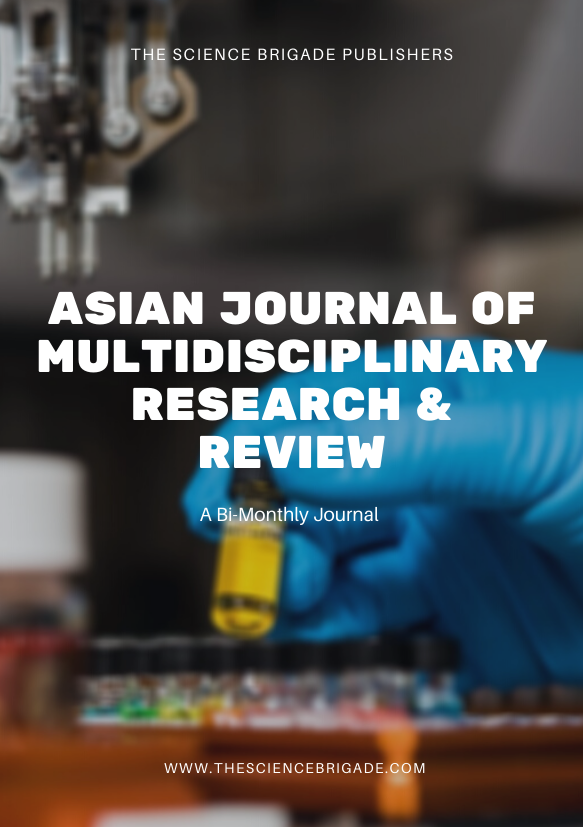
License Information
Copyright © [hfe_current_year]
Danny Jhonson & Jane Smith
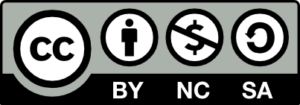
Ownership and Licensing:
Authors of this research paper submitted to the Journal of Science & Technology retain the copyright of their work while granting the journal certain rights. Authors maintain ownership of the copyright and have granted the journal a right of first publication. Simultaneously, authors agreed to license their research papers under the Creative Commons Attribution-NonCommercial-ShareAlike 4.0 International (CC BY-NC-SA 4.0) License.
License Permissions:
Under the CC BY-NC-SA 4.0 License, others are permitted to share and adapt the work, as long as proper attribution is given to the authors and acknowledgement is made of the initial publication in the Journal of Science & Technology. This license allows for the broad dissemination and utilization of research papers.
Additional Distribution Arrangements:
Authors are free to enter into separate contractual arrangements for the non-exclusive distribution of the journal’s published version of the work. This may include posting the work to institutional repositories, publishing it in journals or books, or other forms of dissemination. In such cases, authors are requested to acknowledge the initial publication of the work in the Journal of Science & Technology.
Online Posting:
Authors are encouraged to share their work online, including in institutional repositories, disciplinary repositories, or on their personal websites. This permission applies both prior to and during the submission process to the Journal of Science & Technology. Online sharing enhances the visibility and accessibility of the research papers.
Responsibility and Liability:
Authors are responsible for ensuring that their research papers do not infringe upon the copyright, privacy, or other rights of any third party. The Journal of Science & Technology and The Science Brigade Publishers disclaim any liability or responsibility for any copyright infringement or violation of third-party rights in the research papers.